XSHELL
PAPER SHELL MADE OF CELL UNIT
ELECTIVE COURSE, FALL '19, CORNELL UNIVERSITY
IN COLLABORATION WITH YU SUN, MENGYI YAN, AND JUNSIK OH
XSHELL
PAPER SHELL MADE OF CELL UNIT
ELECTIVE COURSE, FALL '19, CORNELL UNIVERSITY
IN COLLABORATION WITH YU SUN, MENGYI YAN, AND JUNSIK OH
This project explores the simplest way of constructing a doubly-curved shell structure - by setting the fabrication method based on planar manufacture, which can be achieved by CNC Routers or laser machines and using sheet materials like chipboard. The cellular unit was mapped and unrolled to planar folding and cutting lines, fabricated through tens of recycling chipboards within 2mm thickness. The whole fabricating process including cutting, folding, and assembling took only 5 days and 3 group members to complete the construction of this human-scale installation on the 3rd floor of Sibley Hall.
This project explores the simplest way of constructing a doubly-curved shell structure - by setting the fabrication method based on planar manufacture, which can be achieved by CNC Routers or laser machines and using sheet materials like chipboard. The cellular unit was mapped and unrolled to planar folding and cutting lines, fabricated through tens of recycling chipboards within 2mm thickness. The whole fabricating process including cutting, folding, and assembling took only 5 days and 3 group members to complete the construction of this human-scale installation on the 3rd floor of Sibley Hall.
HYDRODYNAMIC WOOD
DESIGN INQUIRY AND MATERIAL CRITIQUE
RESEARCH STUDIO, SUMMER '19, CORNELL UNIVERSITY
IN COLLABORATION WITH RUOCHEN ZHANG, SHIYU JIN, AND PENG ZHANG
This research explores the potential of controlling the deformation of wood material through laminating and moisture adapting. It starts with a deep investigation into the mechanical properties of different wood types, as well as the state-of-art research of fabrication and actuation. A multi-layer lamination approach based on those properties is proposed in accompany by a complete manufacturing workflow integrating AI detection and machine learning. The aggregation of the active-bending behavior is looked through different scales, resulting in both bottom-up and top-down design approaches.

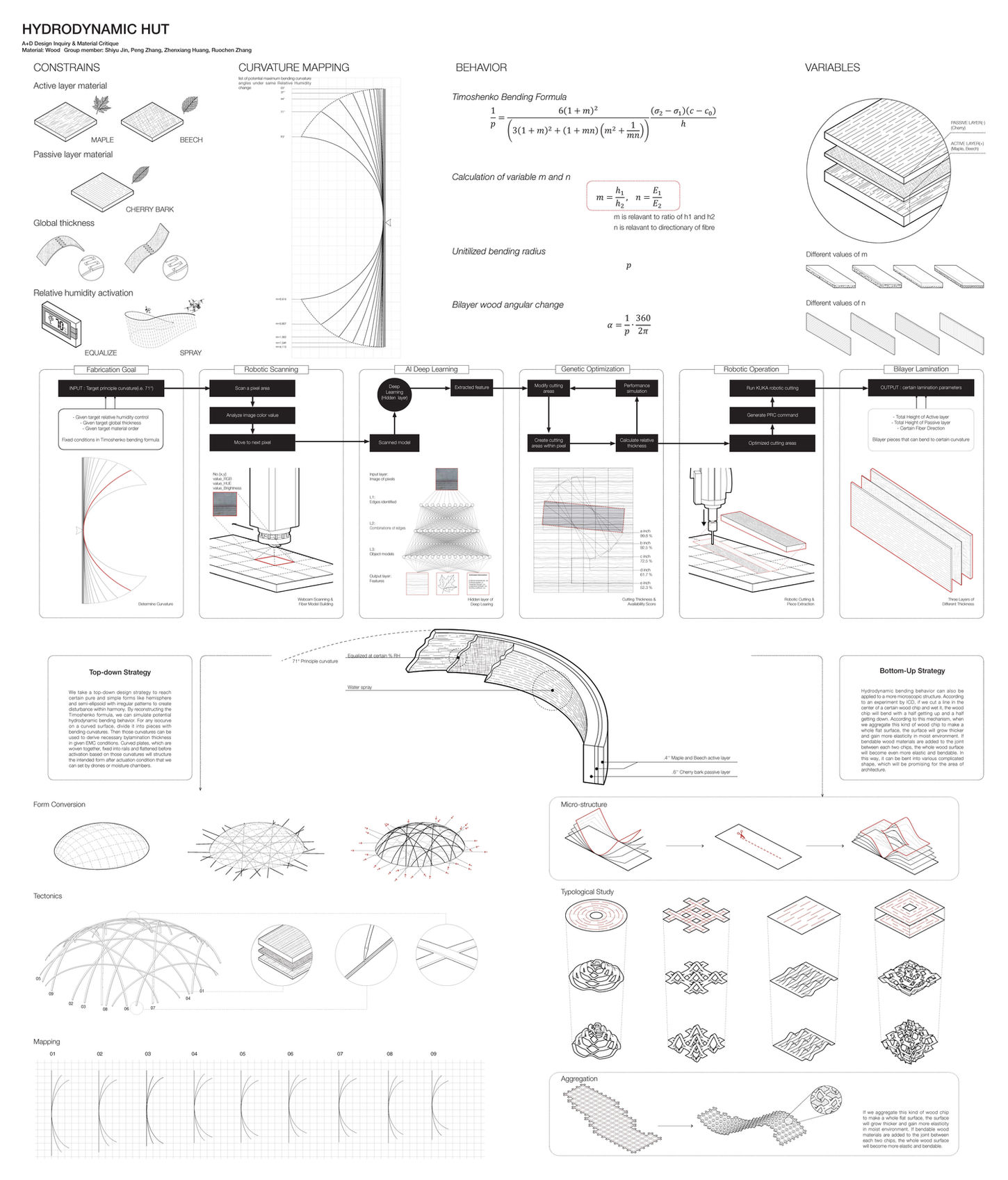
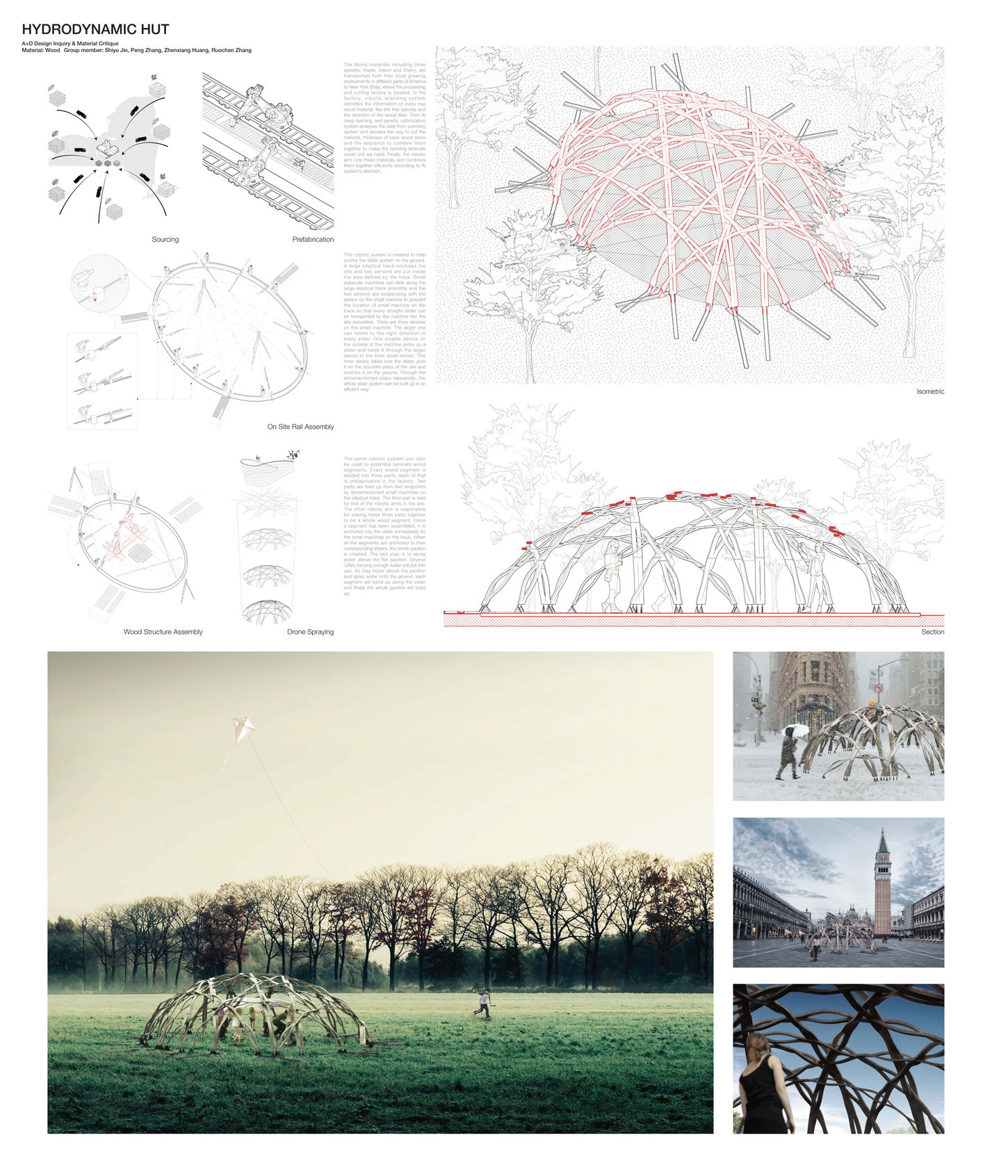
